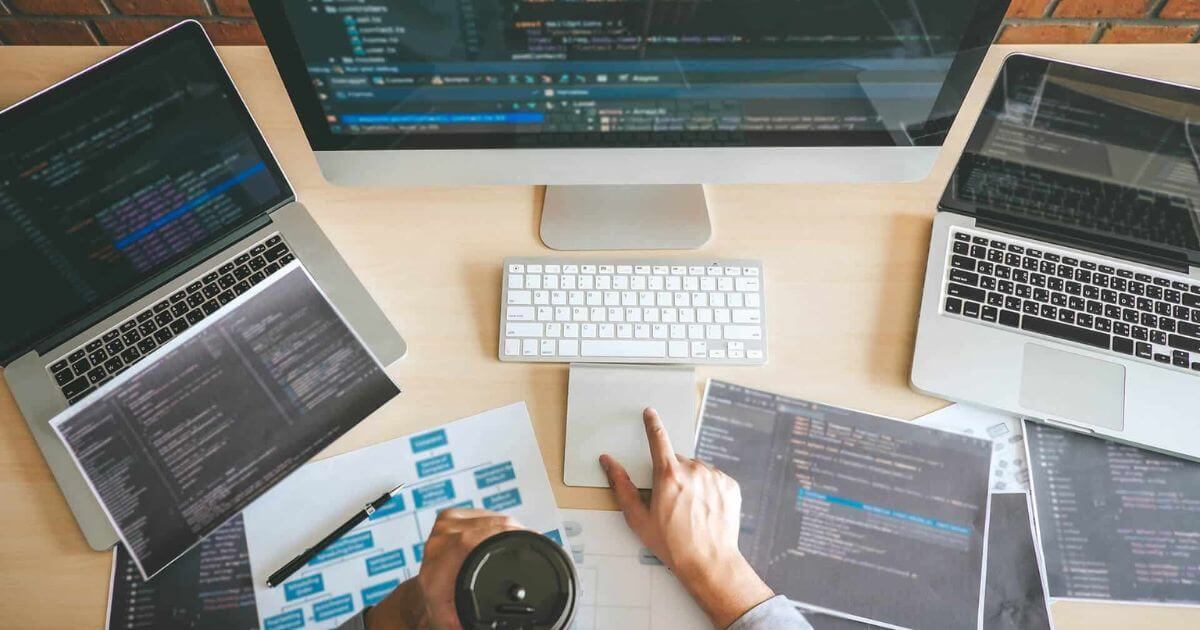
Confidence Interval Calculator
Confidence Interval Calculator
Have you ever wondered if your sample data truly represents the broader population? A Confidence Interval Calculator can provide clarity, offering a statistically sound range where the true population parameter likely falls. By understanding how to effectively use this tool, you'll be better equipped to make informed decisions in your field. It raises important questions about the assumptions we make when interpreting data and the potential impact on your outcomes. What implications could these insights have for your work?
What Is a Confidence Interval?
A confidence interval is a statistical range that estimates the true value of a population parameter, like a mean or proportion, based on sample data.
When you conduct a survey or experiment, you rarely gather data from the entire population. Instead, you collect a sample and use it to make inferences about the whole group. The confidence interval gives you a range where you can reasonably expect the true parameter to lie.
For example, if you calculate a 95% confidence interval for a mean as 50 to 60, it means you can be 95% confident that the actual mean of the population falls between those two values. This range is influenced by your sample size; larger samples typically yield narrower intervals, indicating more precise estimates.
Moreover, confidence intervals aren't definitive. They provide a certain level of uncertainty and variability inherent in sampling. You might encounter different intervals if you draw different samples from the same population.
Understanding what a confidence interval represents helps you gauge the reliability of your estimations, ultimately leading to more informed decision-making in your analysis.
Importance of Confidence Intervals
Confidence intervals play an important role in data analysis and decision-making. When you're working with sample data, it's vital to understand the uncertainty surrounding your estimates. Confidence intervals provide a range of values that likely contain the true population parameter, helping you gauge the precision of your results. By using confidence intervals, you can make more informed decisions.
Instead of relying solely on point estimates, which can be misleading, you've got a range that indicates variability. This helps in evaluating risk and making predictions. For example, if you're examining a new product, knowing the confidence interval of projected sales can guide your marketing strategy. Additionally, utilizing tools like a Confidence Interval Calculator can streamline your calculations and guarantee accuracy.
Moreover, confidence intervals enhance the credibility of your analysis. They show that you recognize the limitations of your data and the inherent uncertainty in your findings. This transparency is essential in fields like healthcare, finance, and social sciences, where data-driven decisions can greatly impact outcomes.
In short, confidence intervals not only aid in interpreting results but also bolster decision-making by incorporating a measure of reliability, making them indispensable tools in your analytical toolkit.
How to Calculate Confidence Intervals
To calculate confidence intervals, you'll first need to understand the components involved, including the sample mean, standard deviation, and the desired confidence level.
Begin by collecting your sample data. Calculate the sample mean (x̄) by summing all your data points and dividing by the number of observations. Next, compute the standard deviation (s) to measure how spread out the data is, using the formula for sample standard deviation.
Once you've got these numbers, decide on your desired confidence level—common choices are 90%, 95%, or 99%. This level indicates how confident you're that the true population parameter lies within your interval.
Now, find the critical value (z or t) corresponding to your confidence level using a z-table or t-table, depending on your sample size.
Multiply your standard deviation by the critical value and divide by the square root of your sample size to get the margin of error (E).
Types of Confidence Intervals
What different types of confidence intervals can you use for your data analysis? The most common types include the normal, t-distribution, and proportion confidence intervals. Each has its own applications depending on your data's characteristics.
The normal confidence interval is used when your sample size is large (generally over 30) or if the population standard deviation is known. It assumes your data follows a normal distribution, providing a straightforward approach to estimating population parameters.
On the other hand, the t-distribution confidence interval is ideal when your sample size is small (below 30) or the population standard deviation is unknown. It accounts for the additional uncertainty by providing wider intervals, which improves the accuracy of your estimates.
Lastly, if you're dealing with proportions, you'd likely use a proportion confidence interval. This type is particularly useful for binary data where you're interested in estimating the proportion of successes in a given sample.
Choosing the right type of confidence interval guarantees that your estimates reflect the underlying data accurately, giving you more reliable insights for your analysis.
Always consider the nature of your data when selecting the appropriate interval.
Common Applications in Research
Understanding the types of confidence intervals sets the stage for their practical use in various research applications. You might find confidence intervals beneficial in fields such as health sciences, social research, and market analysis.
For instance, when conducting a clinical trial, you can use confidence intervals to estimate the effect of a new medication. A narrow interval can boost confidence in the results, helping you determine if the treatment is effective.
In social sciences, you'll often apply confidence intervals to survey data. By calculating these intervals, you can estimate how representative your sample is of the overall population, allowing for more accurate generalizations. This is vital for reporting findings in studies.
In market research, confidence intervals let you assess consumer preferences better. If you're surveying customer satisfaction, you'll want to know how confident you can be in your results.
With confidence intervals, you can provide stakeholders with a clearer picture of potential customer responses.
Factors Affecting Confidence Intervals
Several factors can greatly influence the width and reliability of confidence intervals, so it's essential to take them into account when analyzing your data.
First, consider the sample size. A larger sample size generally leads to a more precise estimate, producing a narrower confidence interval. When you gather more data, you'll reduce variability and enhance your estimate's accuracy.
Next, think about the variability within your data. Higher variability will widen the confidence interval, while lower variability will narrow it. If your data points are more spread out, you'll need a wider interval to capture the true population parameter.
The confidence level you choose also plays a significant role. Common choices are 90%, 95%, or 99%. A higher confidence level requires a wider interval to guarantee that the true parameter is captured within that range.
Tools for Calculating Confidence Intervals
When it comes to calculating confidence intervals, various tools are at your disposal that can simplify the process and enhance your accuracy. You can use statistical software like R or Python, which offer built-in functions for calculating confidence intervals based on your dataset. Both platforms allow for flexibility and customization, enabling you to adapt the calculations to specific needs.
For those who prefer a more user-friendly experience, online calculators are available. These calculators typically require you to input your sample data, the desired confidence level, and a few simple parameters. In seconds, you'll have your confidence interval.
If you're working with spreadsheets, Excel and Google Sheets provide functionalities to compute confidence intervals. You can use formulas like CONFIDENCE or CONFIDENCE.T, making it easy to obtain results directly within your datasets.
Lastly, various statistical textbooks and resources provide guidelines to manually calculate confidence intervals if you prefer a hands-on approach.
Whether you choose software, online calculators, or manual calculations, selecting the right tool will help streamline your work and guarantee your confidence intervals are accurate.
Conclusion
In summary, understanding confidence intervals is like having a safety net when diving into data. They provide an essential cushion, helping you grasp where a population parameter likely falls. With the right tools and knowledge, you can confidently navigate through research, ensuring your decisions are well-informed. So, whether you're exploring health trends or market insights, keep confidence intervals in your toolkit to illuminate the path ahead. Trust the numbers, and let them guide your findings!